Factor Analysis
Identifying the Principal Components of Risk Perception
I conducted a survey using a random sample of online participants. In this survey, I asked respondents to rate several risky behaviors on two sets of process variables: internal and external. Internal variables are those related to the individual (i.e., self-assessed measures that suggest some level of self-agency over the risk being evaluated), while external variables are defined as those related to the risk itself (e.g., probability of a negative outcome) or the environment in which the risk is occurring (e.g., severity). Principal component factor analysis was completed to determine if any of the internal or external variables should be combined into a single measure.
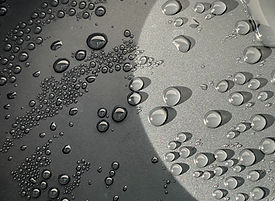
Analysis
In an online survey, I measured several variables related to different risky behaviors (e.g., riding a bicycle without a helmet, investing 10% of one's income in a speculative stock) across various domains (e.g., financial, health/safety, ethical, social, and recreational). The variables measured were: knowledge, control, familiarity, expertise, dread, severity, probability of a positive outcome, and probability of a negative outcome. The variables were categorized based on whether they were internal (related to the individual) or external (related to the risk or environment in which the risk was occurring). The internal variables were knowledge, control, familiarity, and expertise. The remaining variables were categorized as external.
​
In order to test whether these measures, or a subset of these measures, should be combined into a single variable, I conducted a factor analysis on the internal variables and a separate factor analysis on the external variables.
​
​
Internal Variables
For the internal variables, I performed a factor analysis with principal components factor estimation, which assumes the commonalities are equal to one. I then used a varimax rotation to maximize the loadings in the pattern matrix. This analysis is shown in Tables 1A and 1B below. From this analysis, two factors are retained. The variables expertise and familiarity have high loadings on the same factor, while knowledge loads highly on the second factor. The LR test for this analysis was significant (χ2(6) = 529.86, p < .001).
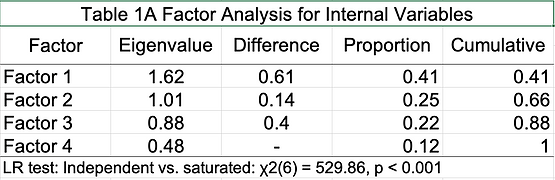
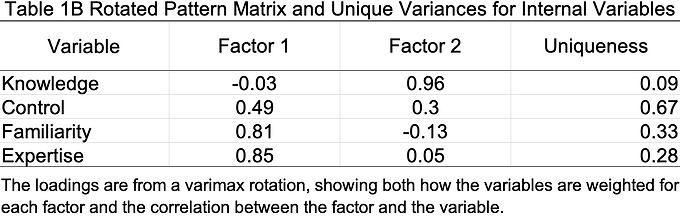
External Variables
For the external variables, I performed a factor analysis with principal components factor estimation. I then used a varimax rotation to maximize the loadings in the pattern matrix. This analysis is shown in Tables 2A and 2B. As the results show, two factors are retained. The variables severity and dread have high loadings on the second factor, while the probability of a negative outcome and probability of a positive outcome have high but opposing loadings on a single factor. The LR test for this analysis was significant (χ2(6) = 975.12, p < .001).
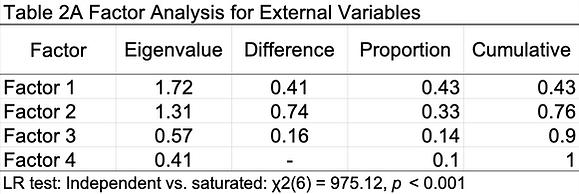

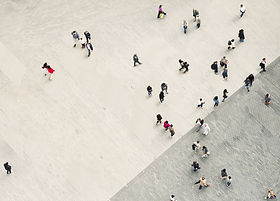
Conclusions
From this analysis, I conclude that the internal variables of knowledge and control should be treated as separate measures, while expertise and familiarity should be combined into a single index (α = 0.67).
For the external variables, I conclude that dread and severity can be combined into one index measure (α = 0.58), while only one of the outcome probability variables should be retained in subsequent analyses.